Resources
That's Fresh! Newsletter
Read a selection of our past issues.
- 🙌 NumPy 2.0 is almost out!And: Our new data preprocessor with Polars | Interview with S2E at Italy Insurance ForumJune 5, 2024
- 😮 What a month for new LLMs!And: Datacamp webinar with ShaliniMay 22, 2024
- ✨ GenAI true value lies beyond operational enhancementsAnd: The Future of Data Protection | New updates about AI ActApril 24, 2024
- 👁 What are 1-bit Large Language Models?And: Linkedin Live about AI Act | Mastercard's Country Manager interviewed our CEOMarch 6, 2024
- LLaMAntino - Effective Text Generation in ItalianAnd: Creating train and test datasets | Use case: Detecting money muling with the help of synthetic dataFebruary 21, 2024
- 🗞️ The NY Times sues OpenAI and MicrosoftAnd: Can AI work with little data? | La Stampa: AI means developmentJanuary 10, 2024
- Synthetic Data 101 🚨And: Why synthetic data? | New project with Poste ItalianeNovember 8, 2023
- How easy is it for LLM to infer sensitive information?And: Why is data sharing important? | Our new partnership with S2EOctober 25, 2023
- Have you heard of Pythia?And: Data augmentation tutorial | Did you say AI apocalypse?August 30, 2023
- Google's answer to ChatGPTAnd: Generating synthetic data within relational databases. Let's meet at WAICF!February 8, 2023
- Understanding ChatGPT betterAnd: How to deal with imbalanced data. More about our productDecember 14, 2022
- A curated list of failed ML projectsAnd: How to build a data strategy. Clearbox AI and Bearing Point partnership.November 16, 2022
- Our open source library is now on GitHubAnd: Clearbox AI on Cybernews.June 22, 2022
- Discovering DagsterAnd: Quantifying privacy risks. Use case: a synthetic data sandbox to freely share data.June 8, 2022
- Can interaction data be fully anonymized?And: Synthetic Data for privacy preservation: understanding privacy risks. Discover our Enterprise solution.April 6, 2022
- What are GFlow nets?And: Improve models with Synthetic Data. Use case: augment financial time series.March 16, 2022
- The European Commission selected us for Women TechEU pilot project!And: What is Synthetic Data. The new Synthetic Data platform.March 09, 2022
- The EDPS on Synthetic DataAnd: From raw to good quality data. Changelogs: now you can upload unlabeled datasets.February 23, 2022
- 2022 Gartner’s Technology TrendsAnd: How to harness the power of AI in companies. Changelogs: new metrics available for your synthetic dataset.February 09, 2022
FROM THE AI WORLD
Today I would like to talk about Pythia, have you heard of it? EleutherAI is a non-profit AI research lab created to replicate and distribute an open-source version of GPT-3. As a result of this effort, they created models such as GPT-J and GPT-Neo, which can be very competitive compared to closed-source solutions. Their research now focuses on large language models' interpretability and alignment properties. They recently released Pythia, a unique set of 16 publicly available large language models designed to foster scientific research on this topic.
The uniqueness of this suite is that the 16 models have been trained with varying sizes and on identical data sequences with intermediate training checkpoints. This structure enables researchers to address gaps in understanding language models' learning dynamics and scaling laws. In the paper, the authors propose different issues that can be investigated thanks to this suite.
For example, they show how modifying the frequency of gendered terms during training can reduce gender bias. The models are available on HuggingFace, making analyzing and experimenting with different model sizes even easier!
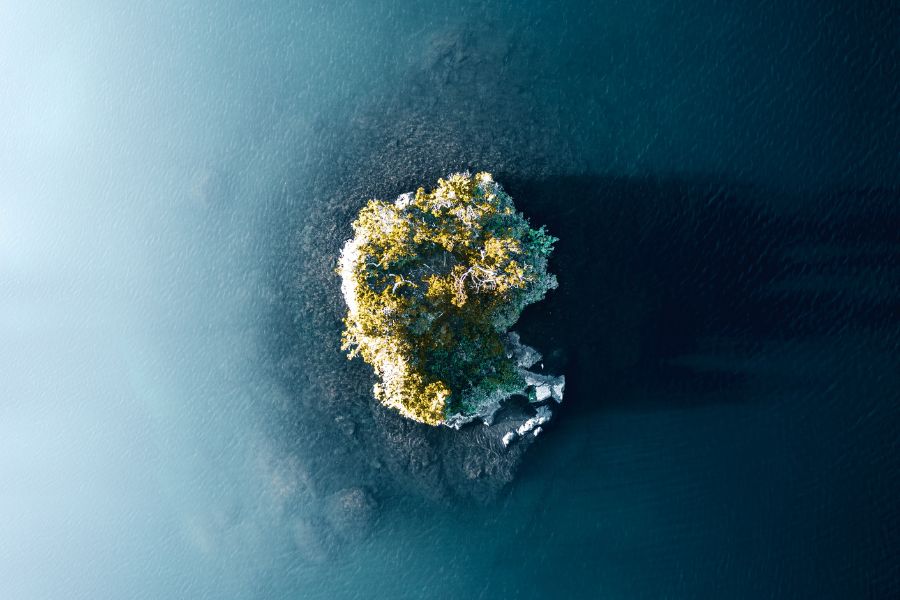
Analyzing LLMs by training and scaling
Pythia combines interpretability analysis and scaling laws to understand how knowledge evolves during training in autoregressive transformers.
CLEARBOX AI
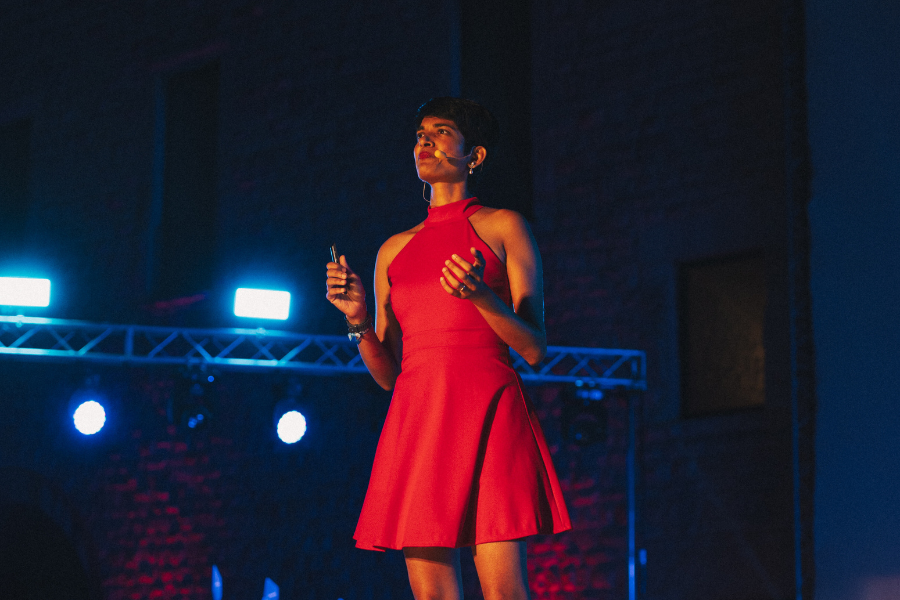
Did you say AI apocalypse?
In her recent TED talk, our CEO Shalini Kurapati discussed the real implications of AI in our society and dispelled the myth of a science-fictional AI apocalypse.
BLOG POST
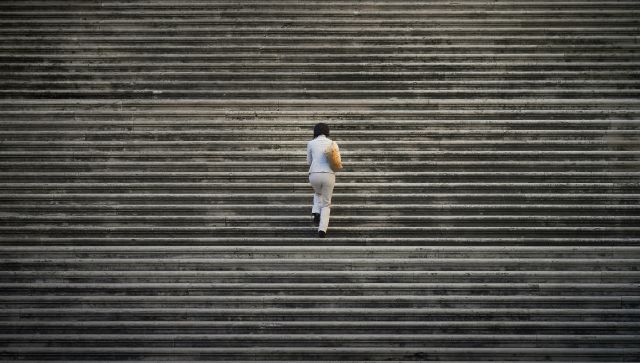
Data augmentation tutorial
Discover how synthetic data can help tackle specific issues affecting datasets that can propagate to poor model performance.
WEEKLY MEME
Your pals may also like...
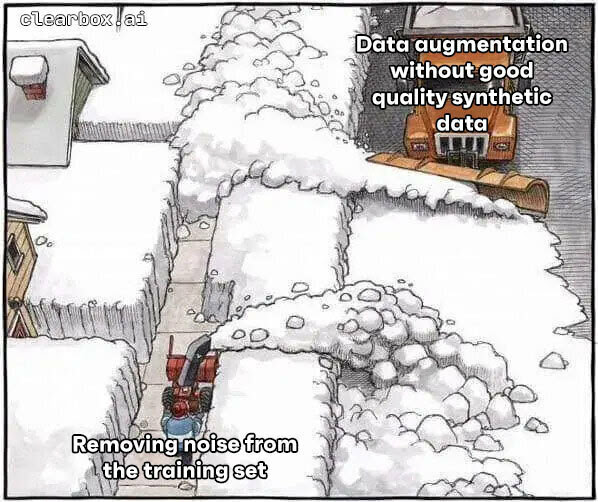