Resources
That's Fresh! Newsletter
Read a selection of our past issues.
- 🙌 NumPy 2.0 is almost out!And: Our new data preprocessor with Polars | Interview with S2E at Italy Insurance ForumJune 5, 2024
- 😮 What a month for new LLMs!And: Datacamp webinar with ShaliniMay 22, 2024
- ✨ GenAI true value lies beyond operational enhancementsAnd: The Future of Data Protection | New updates about AI ActApril 24, 2024
- 👁 What are 1-bit Large Language Models?And: Linkedin Live about AI Act | Mastercard's Country Manager interviewed our CEOMarch 6, 2024
- LLaMAntino - Effective Text Generation in ItalianAnd: Creating train and test datasets | Use case: Detecting money muling with the help of synthetic dataFebruary 21, 2024
- 🗞️ The NY Times sues OpenAI and MicrosoftAnd: Can AI work with little data? | La Stampa: AI means developmentJanuary 10, 2024
- Synthetic Data 101 🚨And: Why synthetic data? | New project with Poste ItalianeNovember 8, 2023
- How easy is it for LLM to infer sensitive information?And: Why is data sharing important? | Our new partnership with S2EOctober 25, 2023
- Have you heard of Pythia?And: Data augmentation tutorial | Did you say AI apocalypse?August 30, 2023
- Google's answer to ChatGPTAnd: Generating synthetic data within relational databases. Let's meet at WAICF!February 8, 2023
- Understanding ChatGPT betterAnd: How to deal with imbalanced data. More about our productDecember 14, 2022
- A curated list of failed ML projectsAnd: How to build a data strategy. Clearbox AI and Bearing Point partnership.November 16, 2022
- Our open source library is now on GitHubAnd: Clearbox AI on Cybernews.June 22, 2022
- Discovering DagsterAnd: Quantifying privacy risks. Use case: a synthetic data sandbox to freely share data.June 8, 2022
- Can interaction data be fully anonymized?And: Synthetic Data for privacy preservation: understanding privacy risks. Discover our Enterprise solution.April 6, 2022
- What are GFlow nets?And: Improve models with Synthetic Data. Use case: augment financial time series.March 16, 2022
- The European Commission selected us for Women TechEU pilot project!And: What is Synthetic Data. The new Synthetic Data platform.March 09, 2022
- The EDPS on Synthetic DataAnd: From raw to good quality data. Changelogs: now you can upload unlabeled datasets.February 23, 2022
- 2022 Gartner’s Technology TrendsAnd: How to harness the power of AI in companies. Changelogs: new metrics available for your synthetic dataset.February 09, 2022
FROM THE AI WORLD
I recently discovered Dagster at a conference and was genuinely impressed by its potential. Dagster is an open-source data orchestration platform, allowing data engineers to develop, test, and deploy data pipelines at scale. It can be seen as an alternative to Airflow and wants to enable developers to write data pipelines using Python.
Data pipelines are defined as Directed Acyclic Graphs (DAGs), where each graph is composed of several ops. Ops are units of computations, and they can be written and tested locally using Python functions. One of the most important ideas behind Dagster is that developers can work on pipelines by starting small and gradually scaling up. It also includes a web interface, Dagit, to visualise graphs and ops better.
What interested me and will probably push me to play with Dagster is that the learning curve doesn’t seem steep, especially when thinking of using the tool for small scale projects!
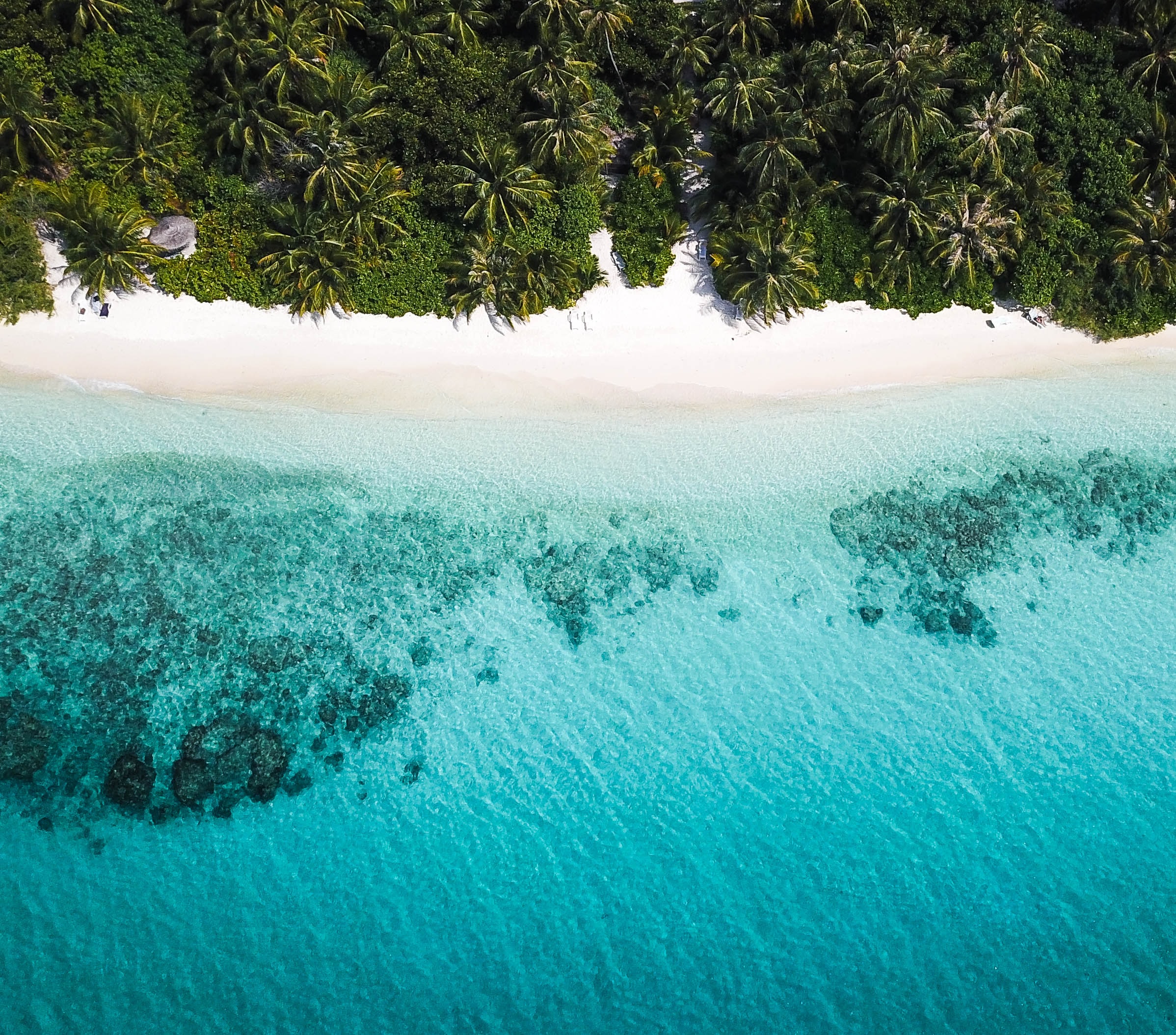
Exploring Dagster platform
This technology is an open-source data orchestration platform for the development, production, and observation of data assets. Do you want to dig deeper?
CLEARBOX AI
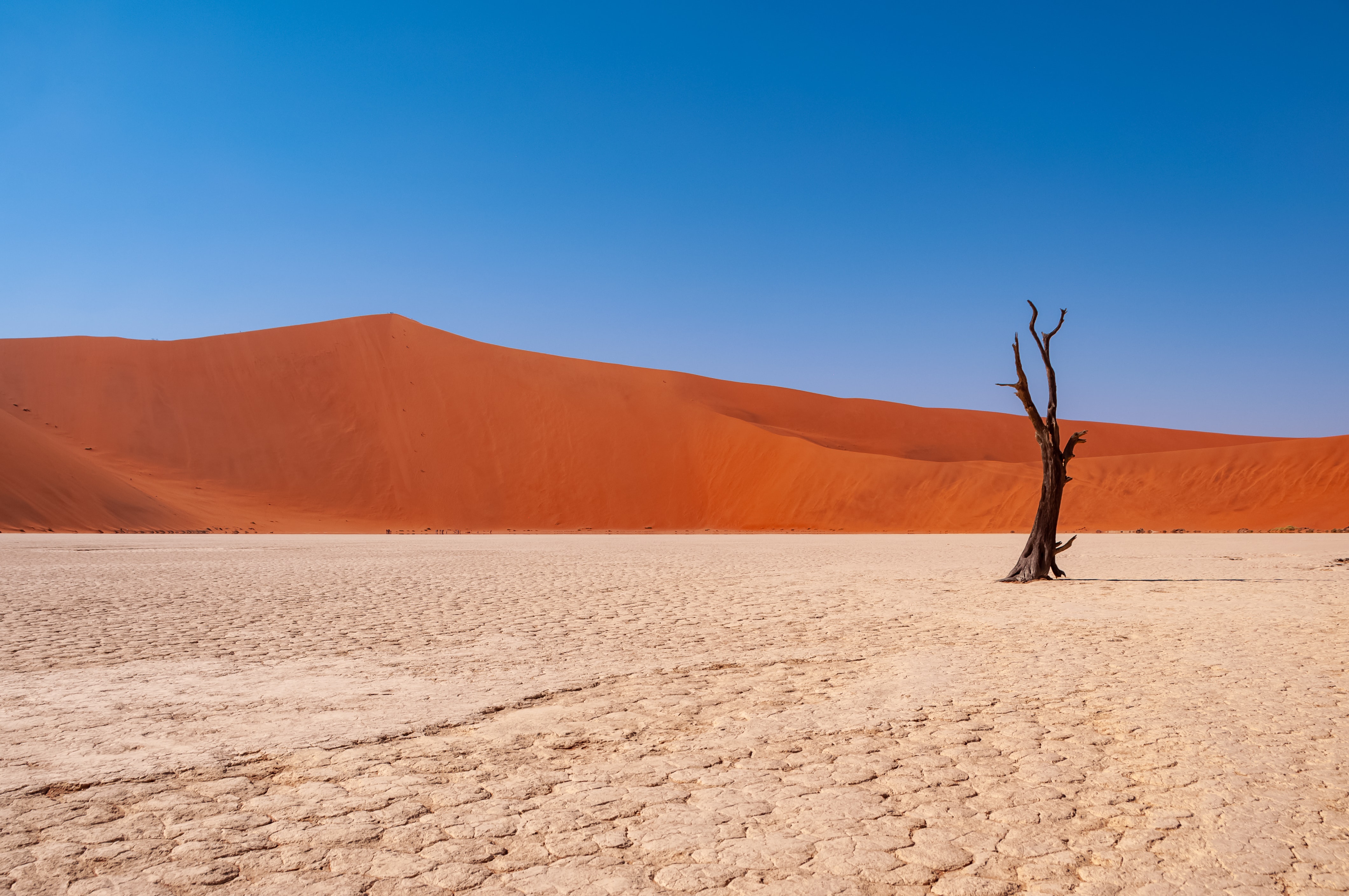
Use case: Synthetic Data sandbox
In this use case we show how our technology is helpful to share and move your data inside and outside your organisation, while complying with privacy regulations.
BLOGPOST
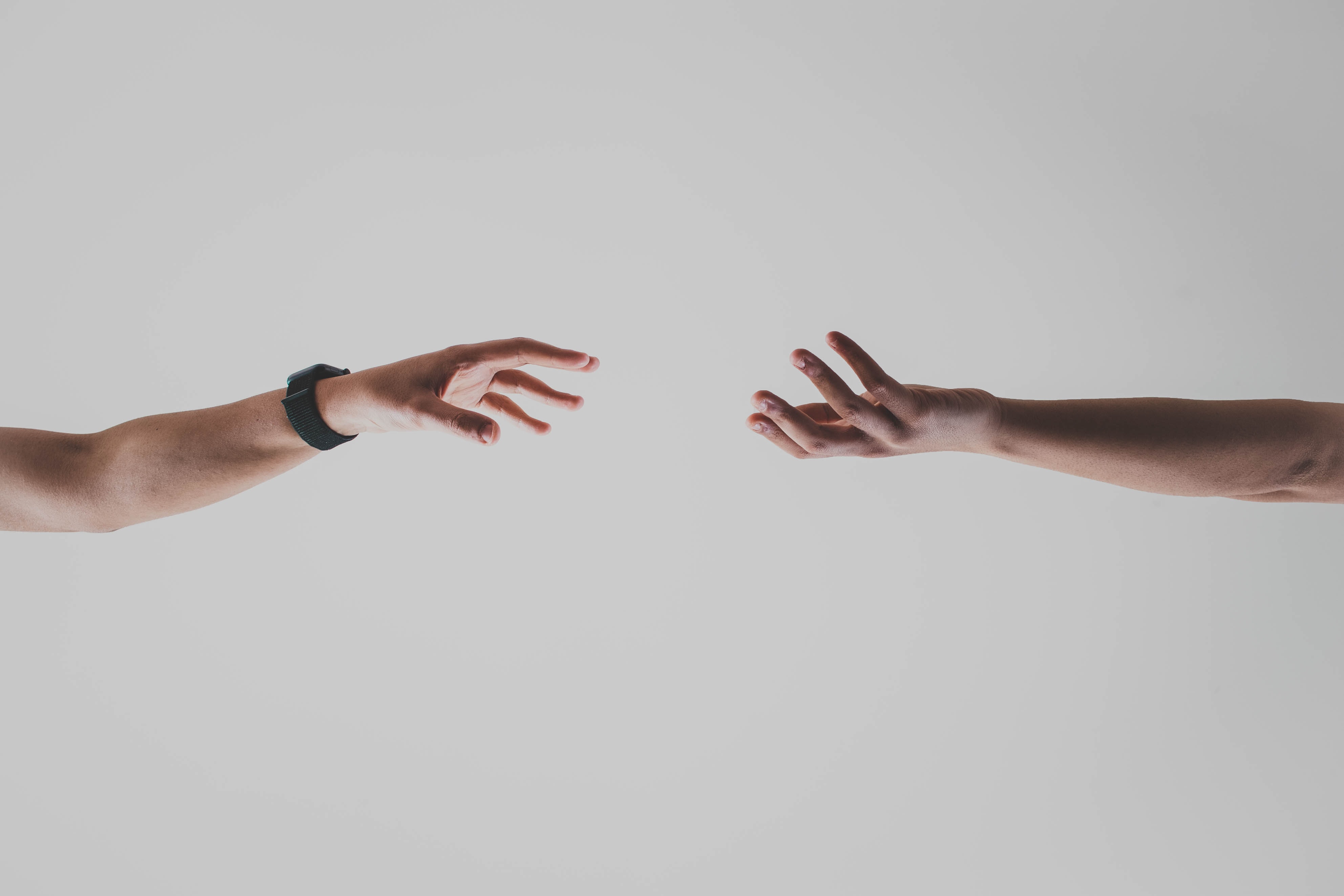
Quantifying privacy risks
In the second part of 'Synthetic Data for privacy preservation' series, our Andrea provides a tutorial on how to quantify and prevent re-identification risk.
WEEKLY MEME
Your pals may also like...
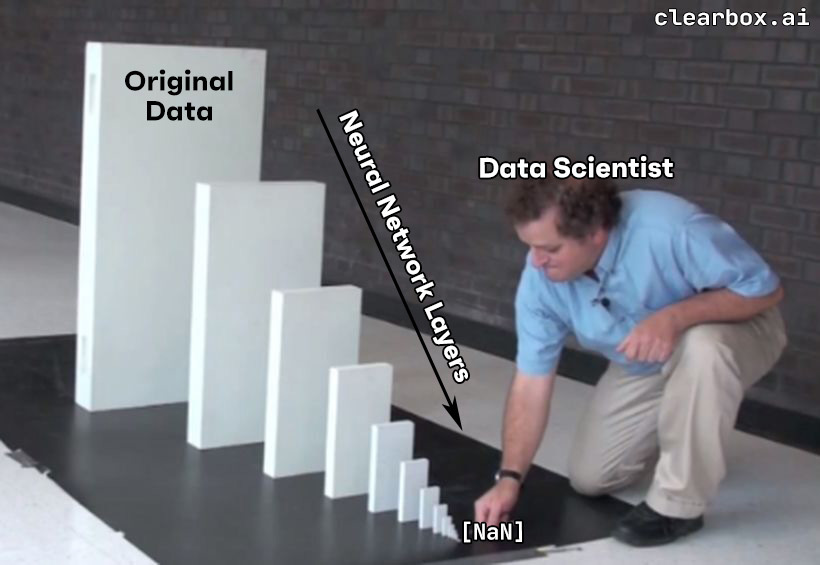