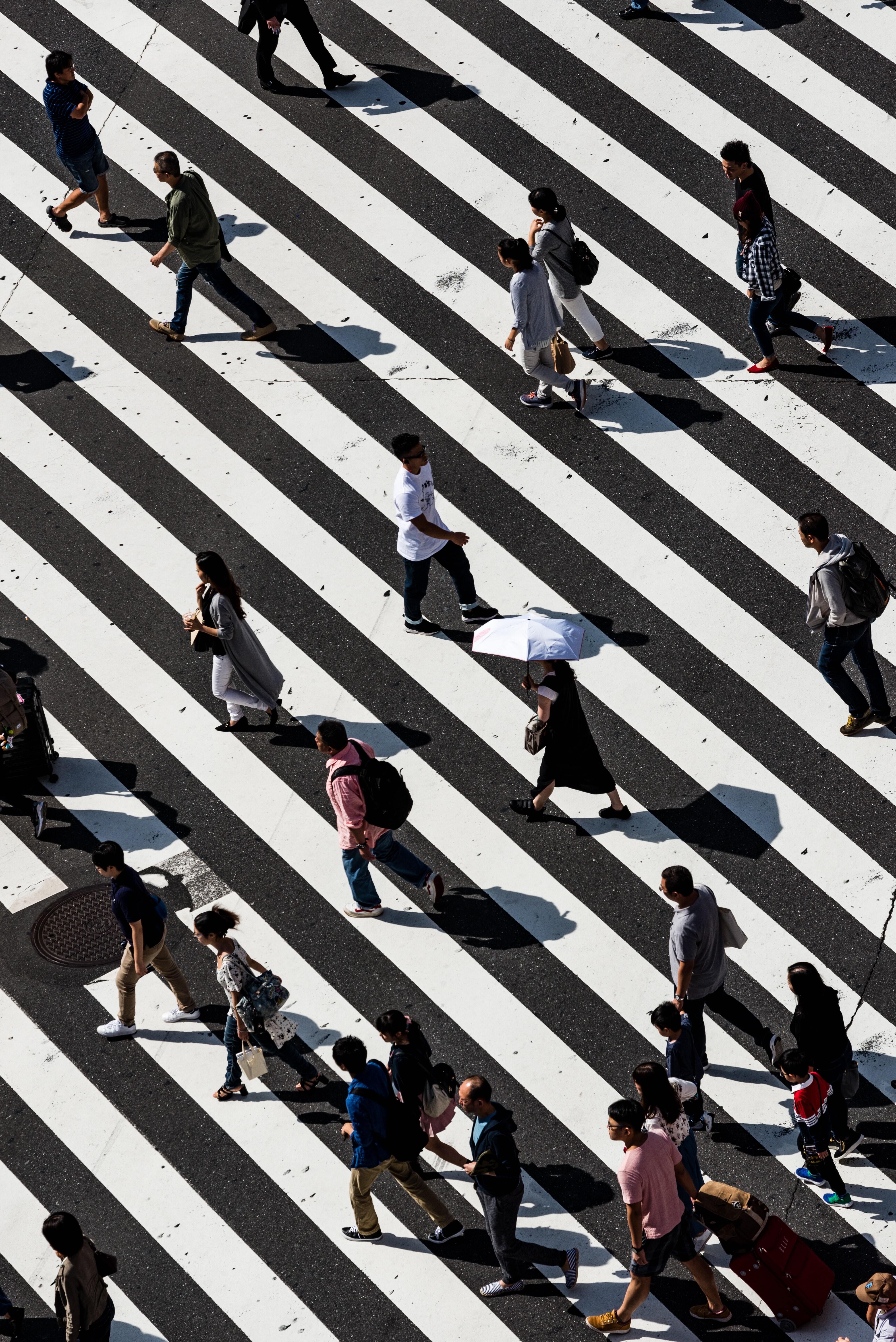
If you are unsure what are the exact differences between anonymization and pseudonymization, you are not alone. We often notice that these two terms are used synonymously with some level of confusion in data privacy conversations. With this blog post, we’d like to clarify these differences in an uncomplicated way.
It’s been more than 4 years since GDPR has become a law and set an international gold standard for data protection, and yet one of the biggest misunderstandings while implementing GDPR is around the concept of anonymization.
First things first, let’s look into their definitions. Researchers and practitioners may interpret the meaning of anonymization and pseudonymization differently based on their respective levels of abstraction and practical implementation. We won’t get into the ideological discussion on that but we will stick to the definitions and requirements within the framework of GDPR.
What is the difference between anonymization and pseudonymization?
According to GDPR:
-
Anonymization is a process that transforms personal data into anonymous data “which does not relate to an identified or identifiable natural person or to personal data rendered anonymous in such a manner that the data subject is not or no longer identifiable”;
-
Pseudonymization is “the processing of personal data in such a manner that the personal data can no longer be attributed to a specific data subject without the use of additional information, provided that such additional information is kept separately and is subject to technical and organisational measures to ensure that the personal data are not attributed to an identified or identifiable natural person”.
To further unpack these high-level definitions- anonymization is the process of removing all personal identifiers, both direct and indirect, that may lead to an individual being identified.
Examples of direct identifiers are name, address, postcode, telephone number, photograph. While, indirect identifiers refer to personal data that gives away identity when with other sources of information, including, place of work, job title, salary, their postcode or health condition.
Whereas, pseudonymization replaces identifying data with made-up values, where the original values are securely kept but can be retrieved and linked back to the pseudonym, should the need arise.
While pseudonymized data falls under GDPR, anonymous data doesn’t. Naturally, organizations strive to make the personal data in their environment anonymous using a variety of methods.
The most common anonymization techniques
Some of the commonly used anonymization techniques in practice are:
-
Aggregation: Data is displayed as totals, so no data relating to or identifying any individual is shown. Small numbers in totals are often suppressed through ‘blurring’ or by being omitted altogether.
-
Data masking: This involves stripping out obvious personal identifiers such as names from a piece of information, to create a data set in which no person identifiers are present.
-
Data Perturbation: the values from the original dataset are modified to be slightly different.
-
Data Swapping/Shuffling: The purpose of swapping is to rearrange data in the dataset such that the individual attribute values are still represented in the dataset, but generally, do not correspond to the original records. This technique is also referred to as shuffling and permutation.
-
Suppression: Record suppression refers to the removal of an entire record in a dataset.
Although these techniques are considered as anonymization techniques, they don’t necessarily produce GDPR ‘approved’ anonymous data.
What GDPR means by anonymous data is that the risk of re-identification of an individual from that data is zero. The trouble with this is that it’s practically impossible to achieve and assure 100% anonymity if one wants to derive an iota of value from the resulting anonymous dataset.
For each of these techniques above, there are documented risks of re-identification like identity disclosure/singling out, linkage, inference etc, some of which we have also discussed in detail in our earlier blog post. Our bet is that, when organizations talk about anonymised data, 9 out of 10 times, they talk about a variant of pseudonymized data. Notwithstanding, completely anonymous data is often desirable since it might offer any utility to organizations who operate in the competitive realm of ‘data is the new oil’. As the well-known Privacy Scholar Paul Ohm put it- “Data can be either useful or perfectly anonymous but never both”.
Synthetic Data as an alternative anonymization technique
This challenge of balancing privacy vs. utility has inspired companies as well as Data Protection Authorities to consider new anonymization techniques such as Synthetic Data as a viable alternative.
Synthetic data can especially be a powerful privacy-preserving technique since it creates new data from a sample set of data that preserves the statistical utility of the sample set but does not recreate any direct identifiers. Moreover, synthetic generation creates data sandboxes that help businesses easily share data inside and outside your organisation. Even though synthetic data may not eliminate all the re-identification risks, we can sufficiently quantify and measure these risks to take responsible and informed decisions on further processing of such data.
That is the crux of our Clearbox AI approach of offering high-quality synthetic data together with a robust analysis of re-identification risk insights, to help data scientists and governance teams to extract the most value out of data while complying with relevant laws.
References
Weitzenboeck, Emily and Lison, Pierre and Cyndecka, Malgorzata Agnieszka and Langford, Malcolm, The GDPR and Unstructured Data: Is Anonymization Possible? (April 7, 2022). International Data Privacy Law, 2022; ipac008, https://doi.org/10.1093/idpl/ipac008
Ohm, P. (2009). Broken promises of privacy: Responding to the surprising failure of anonymization. UCLA l. Rev., 57, 1701.
https://www.aepd.es/es/documento/10-anonymisation-misunderstandings.pdf
Personal Data Protection Commission (“PDPC”) Singapore report on “Guide to Basic Anonymisation Techniques” (2018)
Tags:
blogpost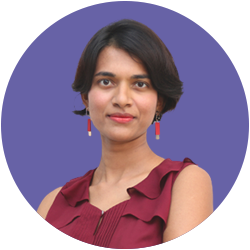