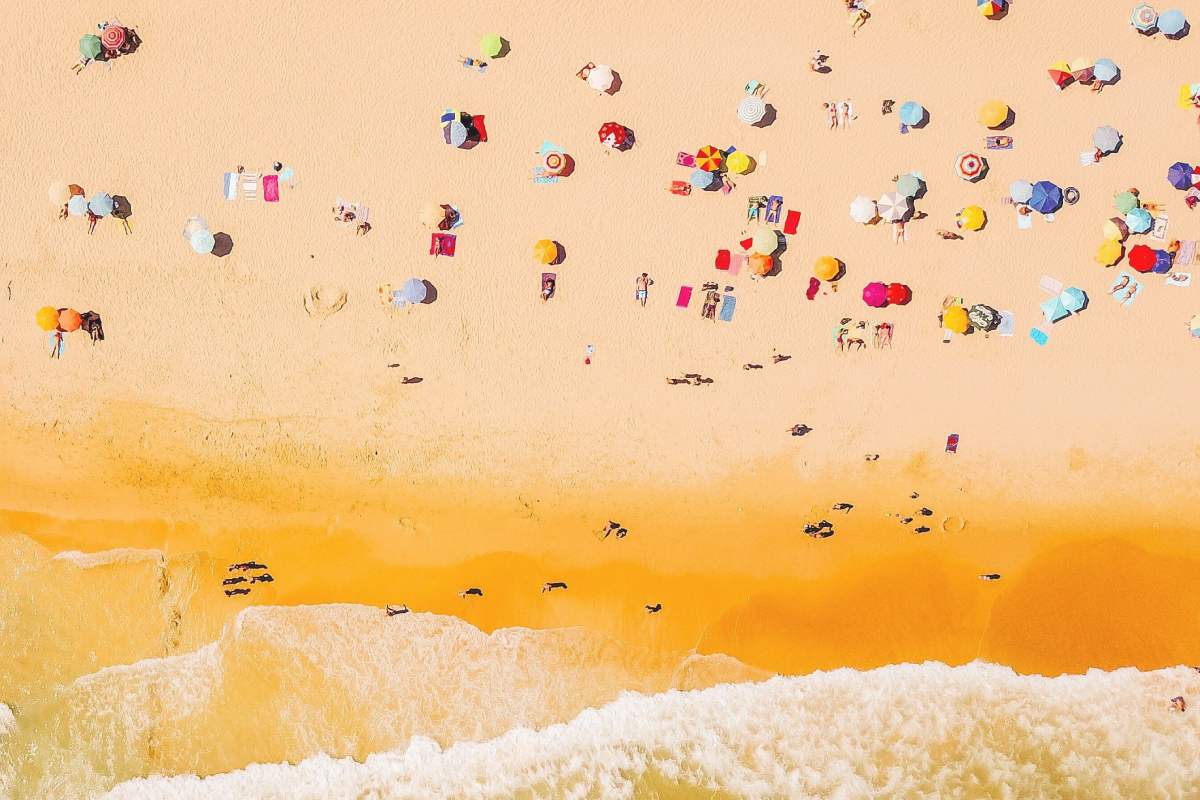
In the last few months we’ve had the chance to engage closely with our first users, and we were able to collect comprehensive feedback and information about their experience with AI Control Room. Our user-centric approach helped us to discover the pain points faced by companies with regards to assessment of the quality of synthetic dataset. Attuning our platform development to this crucial market need, we’ve decided to focus our efforts on a specific module that not only generates synthetic data but also provides a report covering metrics to easily and efficiently assess its quality. So good news, from now on you can try this module yourself in the new version of the AI Control Room!
If this information intrigues you, stop right here and jump right into the platform to get a sneak peek into the look and features of the report. If you want to be convinced even more, continue reading about why data quality assessment of synthetic data is crucial and befitting the data-centric AI approach that motivates our entrepreneurial adventure.
What is a Synthetic Data Quality report and why it is important
The synthetic data quality report contains the results of a series of tests that are run to make sure that the statistical properties and the predictive content of the original dataset are preserved during the generation process. Generating synthetic data could be done in a multitude of ways with varying results, however generating robust and good synthetic data is different and it is not trivial to discriminate between different solutions and results. If we want to repurpose synthetic data for tasks such as testing, data analytics and model training we really need to make sure that the information loss happening within the synthetisation process is minimal.
What’s inside Synthetic Data Quality report
The new data quality report contains the results of the following tests.
Univariate distributions and feature correlation
The first check we can perform when comparing synthetic datasets with respect to their original form is to make sure that statistical distributions are preserved. This is done by comparing univariate distributions and how statistical correlation between features are preserved.
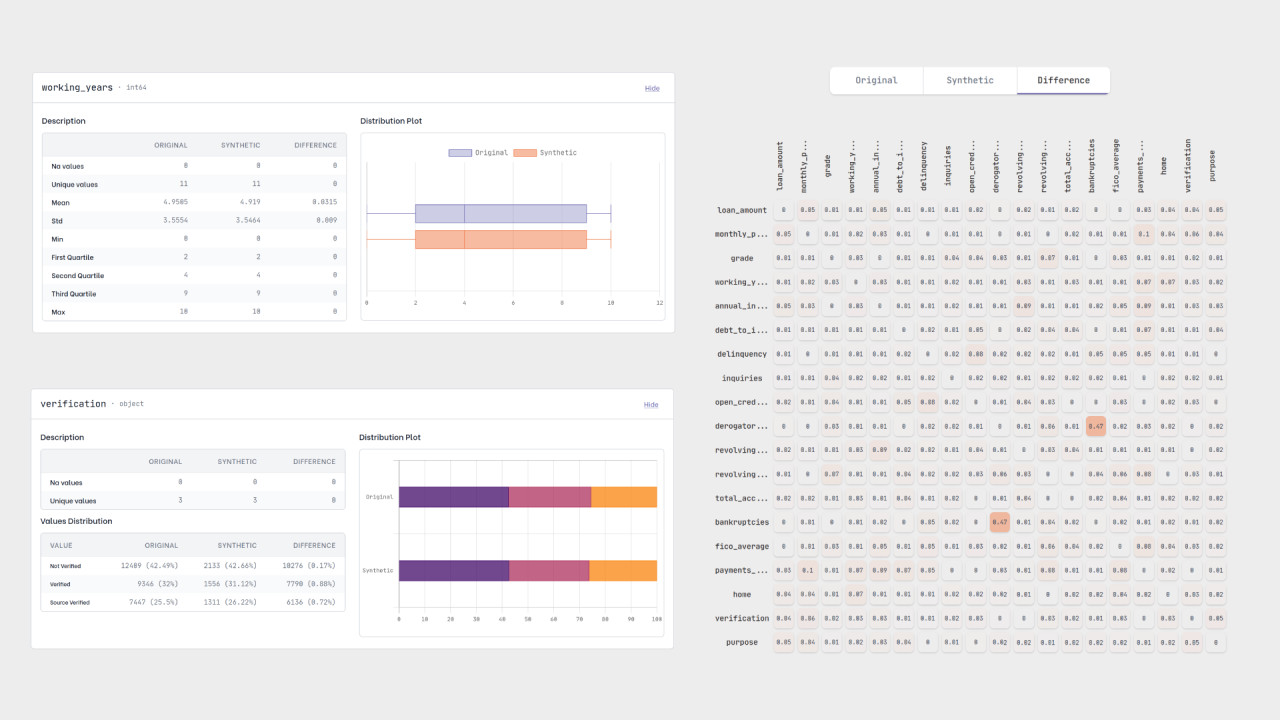
Segment distributions
Our engine performs an unsupervised segmentation of the original dataset. The same segmentation model is applied to the synthetic dataset to make sure points are similarly distributed across different segments.
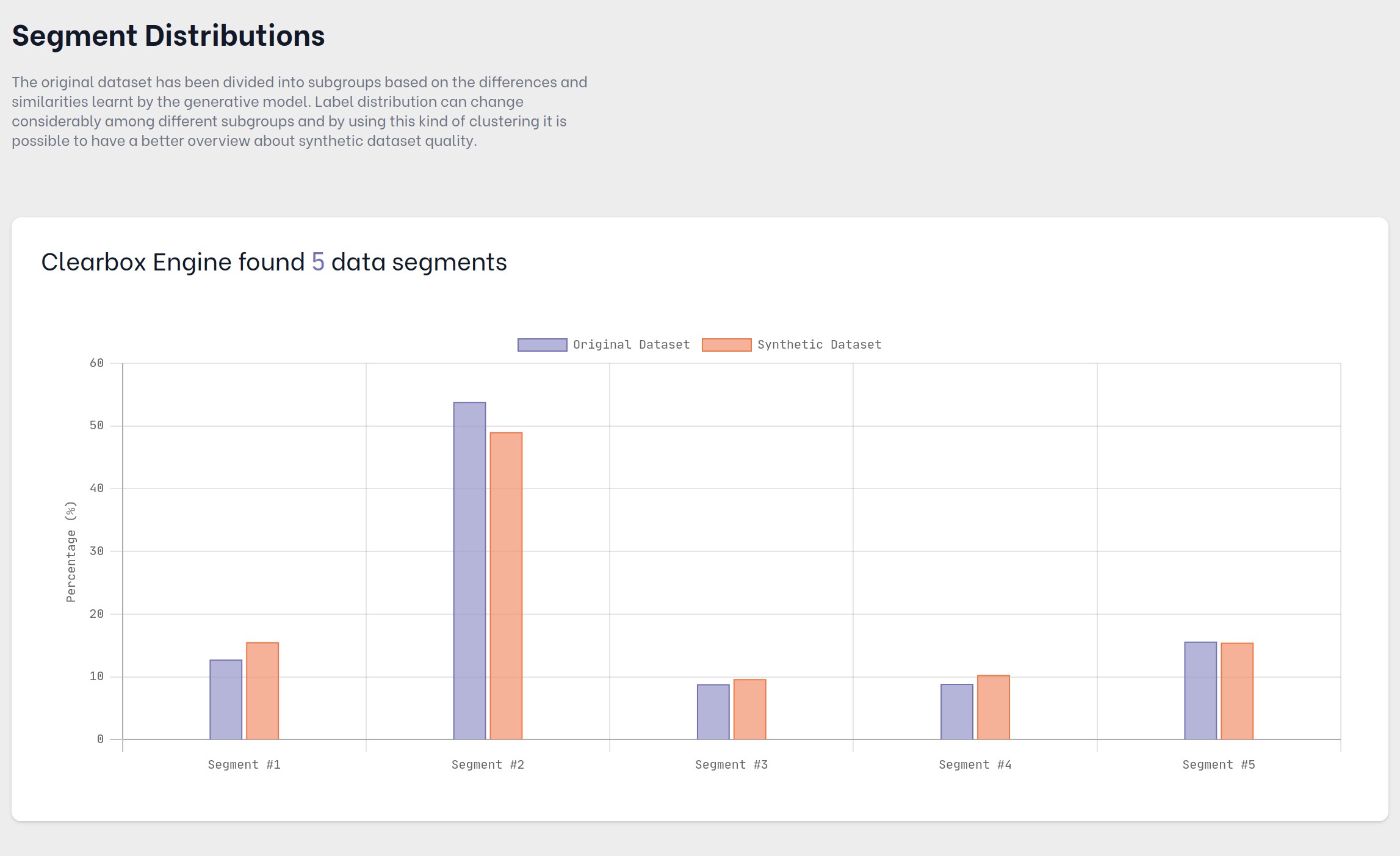
Train on Synthetic Test on Real
Whether we are using synthetic data to train new machine learning models or just to perform data analytics we need to make sure as little information as possible is lost during the cloning process. A very robust test to quantify such information loss is to train a machine learning model on the synthetic dataset and to test it on a hold-out dataset from the original distribution. This model performance can be then compared to the performance of the same model trained on the original dataset.
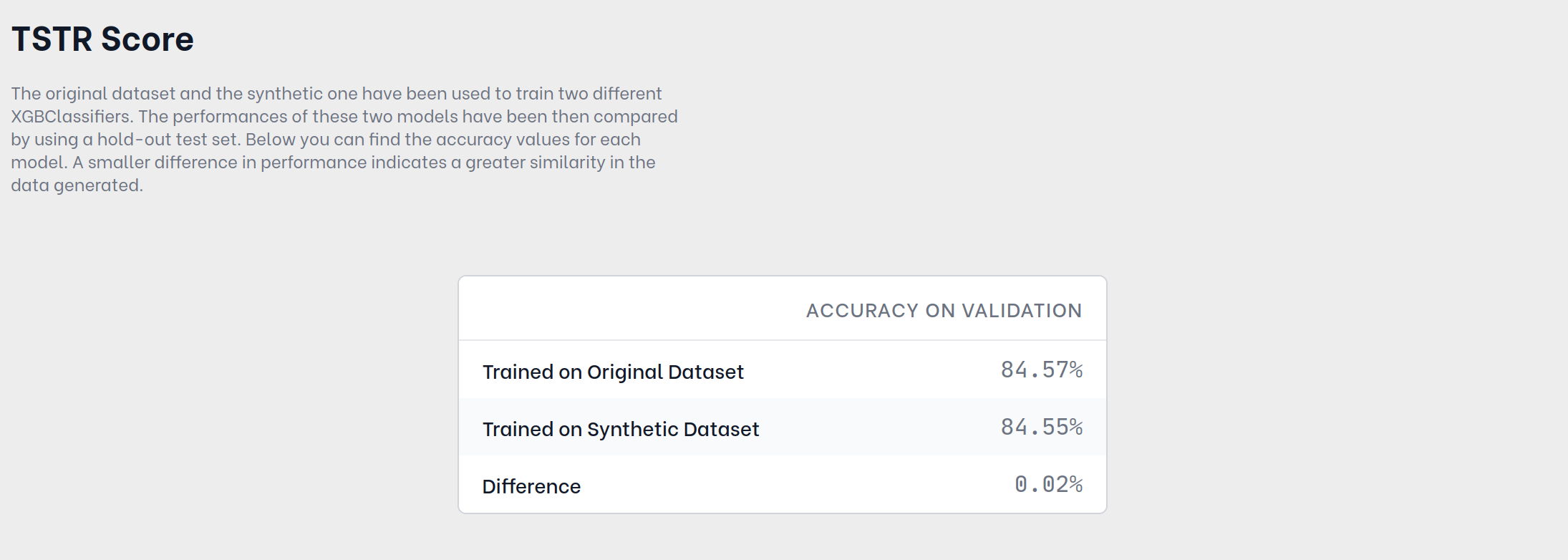
You can quickly jump to AI Control Room and generate synthetic data and data quality reports for free, we look forward to hearing your feedback!
Tags:
news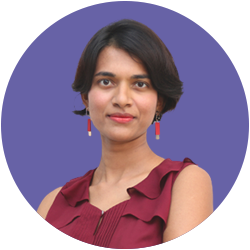